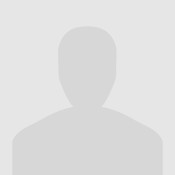
Ahmed A. Elngar
مطالب
Comparing the Performance of Pre-trained Deep Learning Models in Object Detection and Recognition(مقاله علمی وزارت علوم)
کلید واژه ها: deep learning Image recognition Object Detection Pre-trained Models
A Deep Learning Based Analysis of the Big Five Personality Traits from Handwriting Samples Using Image Processing(مقاله علمی وزارت علوم)
کلید واژه ها: computer vision Convolutional neural networks Artificial Neural Networks Machine Learning Big Five Personality Traits Handwriting Graphology
Feature Selection and Hyper-parameter Tuning Technique using Neural Network for Stock Market Prediction(مقاله علمی وزارت علوم)
کلید واژه ها: Neural Network Stock market prediction Numerai NMR deep learning
Guest Editorial: Deep Learning for Visual Information Analytics and Management(مقاله علمی وزارت علوم)
کلید واژه ها: deep learning Visual information Data analytics Watermarking